Neural networks with Flax#
Flax is library for building neural networks with JAX backend. It provides high-level APIs that make it easy to define, train, and deploy neural network models.
In this lecture, we will:
Generate some simulated data,
Define a simple feed-forward neural network using Flax.
Train the model on the simulated data.
Evaluate the trained model’s performance.
import jax
import jax.numpy as jnp
from flax import linen as nn
from flax.training import train_state
import optax
import matplotlib.pyplot as plt
# simulate data
num_samples = 1000
key = jax.random.PRNGKey(42)
X = jax.random.normal(key, shape=(num_samples, 10))
w = jnp.ones((10,))
b = 5.0
noise = jax.random.normal(key, shape=(num_samples,))
y = jnp.dot(X, w) + b + noise
# split into training and test sets
train_X = X[:800]
train_y = y[:800]
test_X = X[800:]
test_y = y[800:]
Defining the Model with nn.Module
#
Let’s define a simple Multi-Layer Perceptron (MLP) model with two hidden layers:
class MLP(nn.Module):
@nn.compact
def __call__(self, x):
# first hidden layer
x = nn.Dense(features=64)(x)
x = nn.relu(x)
# second hidden layer
x = nn.Dense(features=32)(x)
x = nn.relu(x)
# output layer
x = nn.Dense(features=1)(x)
return x
Create training state#
To manage the training process, we need to initialize our model parameters and an optimizer state. We can do this by creating a TrainState
object from Flax.
def init_model(key, input_shape):
model = MLP()
variables = model.init(key, jnp.ones(input_shape))
tx = optax.adam(learning_rate=0.001)
return train_state.TrainState.create(
apply_fn=model.apply,
params=variables['params'],
tx=tx
)
input_shape = (1,) + train_X.shape[1:]
rng_key = jax.random.PRNGKey(12345)
state = init_model(rng_key, input_shape)
Define loss function and update function#
Now, we define the loss function and update function for training the model.
def compute_loss(params, batch):
inputs, targets = batch
preds = state.apply_fn({'params': params}, inputs)
loss = jnp.mean((preds - targets)**2)
return loss
@jax.jit
def train_step(state, batch):
grads = jax.grad(compute_loss)(state.params, batch)
return state.apply_gradients(grads=grads)
Training loop#
Finally, we write the main training loop where we iterate over batches of data and update the model’s parameters.
batch_size = 32
num_epochs = 100
training_losses = []
test_losses = []
for epoch in range(num_epochs):
perm = jax.random.permutation(jax.random.PRNGKey(epoch), len(train_X))
train_X_perm = train_X[perm]
train_y_perm = train_y[perm]
for i in range(0, len(train_X), batch_size):
batch_X = train_X_perm[i:i+batch_size]
batch_y = train_y_perm[i:i+batch_size]
state = train_step(state, (batch_X, batch_y))
# Compute training loss
train_loss = compute_loss(state.params, (train_X, train_y))
training_losses.append(train_loss)
# Compute test loss
test_loss = compute_loss(state.params, (test_X, test_y))
test_losses.append(test_loss)
if epoch % 10 == 0:
print(f"Epoch {epoch}: Training Loss = {train_loss:.4f} | Test Loss = {test_loss:.4f}")
# plotting the training and test losses
plt.figure(figsize=(4, 3))
plt.plot(training_losses, label='Training Loss')
plt.plot(test_losses, label='Test Loss')
plt.xlabel('Epochs')
plt.ylabel('Loss')
plt.title('Training and Test Losses Over Epochs')
plt.legend()
plt.grid(True)
plt.show()
Epoch 0: Training Loss = 21.0659 | Test Loss = 20.1596
Epoch 10: Training Loss = 11.7775 | Test Loss = 12.2506
Epoch 20: Training Loss = 11.5257 | Test Loss = 11.9511
Epoch 30: Training Loss = 11.3783 | Test Loss = 11.7627
Epoch 40: Training Loss = 11.2136 | Test Loss = 11.6640
Epoch 50: Training Loss = 11.1206 | Test Loss = 11.5351
Epoch 60: Training Loss = 11.0571 | Test Loss = 11.4719
Epoch 70: Training Loss = 11.0151 | Test Loss = 11.3930
Epoch 80: Training Loss = 10.9865 | Test Loss = 11.3350
Epoch 90: Training Loss = 10.9756 | Test Loss = 11.3486
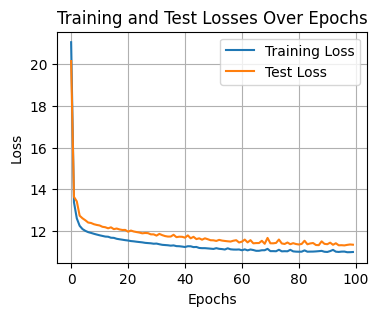